Introduction
Google recently launched its new AI model, Gemini, which responds to text, audio, video, and code. Its ability to interact with the world using text, sound, video, and touch has created a sensation. So, let us understand Gemini and know more about it.
What is Gemini?
Gemini is Google’s most capable and general model with state-of-the-art performance optimized for three sizes: Ultra, Pro, and Nano. People can communicate with Gemini through mixed channels like audio, video, text, and images, making it multimodal.
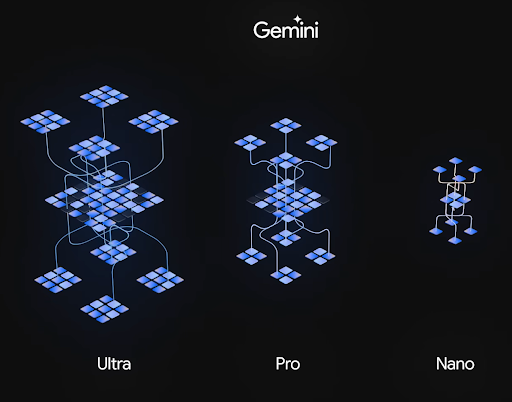
Is Gemini better than ChatGPT?
According to performance reports, it beats OpenAI’s ChatGPT 3.5 and is the first model to outperform human experts on MMLU (massive multitask language understanding). The MMLU consists of questions from 57 subjects, including STEM, humanities, law, medicine, and ethics. In the MMLU benchmark, Gemini Ultra’s performance was 90%, while GPT-4 scored 86.4%.
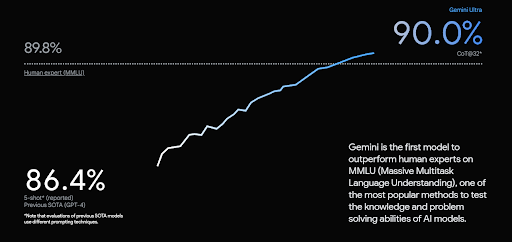
Capabilities
Gemini can produce code from various inputs provided to it. For example, you can feed it a video and ask for ideas, demos, or even an explanation of musical notes. For example, you can share a pic of the available decoration items and a picture of your room and ask for suggestions on how to decorate.
The Potential of Gemini
Boosting scientific literature
Finding and using data extracted from the scientific literature is a common problem scientists face. This standard practice is time-consuming as they need to search thousands of research papers and hand-pick information. Gemini can be used in such cases using proper and exact prompts. Suppose there’s an existing dataset until 2018, and one needs to update it with the latest data until 2023. They can use Gemini to do that by breaking down the procedure and taking its help step-by-step.
First, they’ll need to provide all the necessary details along with a detailed and exact prompt to ask Gemini to provide them with the relevance to their research topic. Second, based on the relevance of the papers, they would give another prompt and extract key data. Gemini also tells where in the research paper it found that information. Finally, running the above steps at scale, one can scan around 200,000 research papers, filter them down to 250, and extract their data in comparatively less time.
Apart from extracting data from text, due to its multimodal nature, we can ask it to refresh our previous findings (let’s say it was in a graph). We can feed our old graph’s image of data until 2018 and ask it to use the newly extracted data and update the line graph.
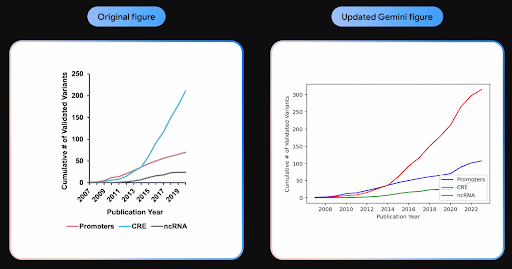
Peer-programming with Gemini
Gemini has improved its solve rate to 75% (from 45% in PaLM 2) in the first attempt. If you ask Gemini to check and repair its mistakes, this percentage goes to 90%, which is a huge jump. Compared to AlphaCode1, which competes with the average human competitor, DeepMind introduced AlphaCode2, powered by Gemini, with massively improved performance. AlphaCode2 is twice as fast as AlphaCode1 and can compete with the top 0.2% of developers to solve superior, strenuous, and complex problems. Developers will now be able to collaborate with AlphaCode and can reason, design, or even help with the actual implementation.
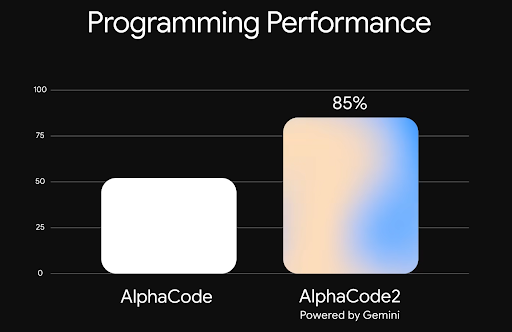
Processing and understanding raw audio signal end-to-end
While processing LLM (large language models), audio is processed by sending it to a speech recognition system, which converts it to text. This converted text is passed on to another model that interprets it. In this method, nuances, like voices and pronunciation, are lost.
Gemini, using its multimodal capabilities, processes the raw audio end-to-end. Furthermore, it can not only process and understand audio nuances but can process it further with other media formats, such as images and text.
Explaining reasoning in math and physics
If you need help with your homework or your kid’s homework, Gemini is here to help. It can read through images, identify math problems, identify mistakes, provide explanations, and help you learn.
Understanding user intentions to create personalized experiences
With Gemini’s multimodal reasoning capabilities, users will get tailored user interfaces beyond traditional chat interfaces for user intents. Gemini first decides whether it should even use a UI. Once the decision to use UI is made and it has enough information and an understanding of the user’s intention, Gemini generates a customized UI. This pattern continues and based on the user inputs, it will follow the same process and decide what type of UI to generate. None of the UI creation is coded and is created based on the requirement.
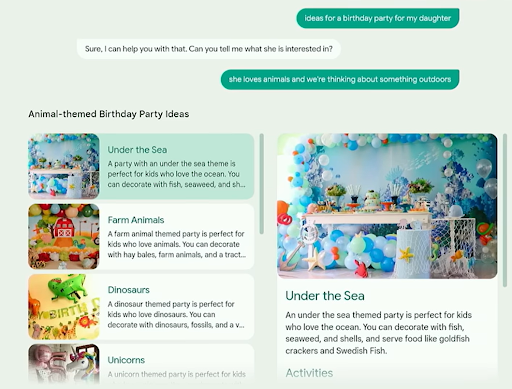
Safety and responsibility
With all the capabilities we read about Gemini come responsibilities and safety concerns. When going from image to text, contextual challenges emerge as an image can be innocuous, but their combination could be offensive or hurtful.
Google developed proactive policies and adapted them to unique multimodal capabilities. These policies allow Gemini to test new risks like cybersecurity and considerations like bias and toxicity. Google is using the red teaming process, involving industrial experts for external evaluations and to get their perspectives on how the model is performing. Furthermore, these models are tested in Google and across the industry. Additionally, Google is creating cross-industry collaborations via frameworks like SAIF (Secure AI framework) to deploy Gemini safely and responsibly.
To sum it up
From turning images into code or finding similarities between two images, Gemini 1.0 can do a lot, you can imagine. It can understand unusual emojis and can even merge them to create new ones! Gemini can help you with your outfits, learn music, or even help interpret musical notes. You can share images of an environment and ask for help revamping it, decorating it, or even asking for ideas to rearchitect it.